Unmanned heavy machinery to work in dangerous environment such as construction sites, disaster areas and the moon has been developed. However, it is still necessary to improve its work efficiency especially during bulldozing and excavating soil. This research aims to develop a method for predicting soil deformation using machine learning. Moreover, to apply the method to planning an optimal orbit to excavate for improving the efficiency. Soil deformation is captured as depth data and then converted to point cloud data for machine learning. Recurrent Neural Network (RNN) is used to develop learned models in this research because soil deformation is continuous phenomena depending on time variation.
Contents
Previous researches
Development of a time-series deformation prediction model for excavated sediment geometry
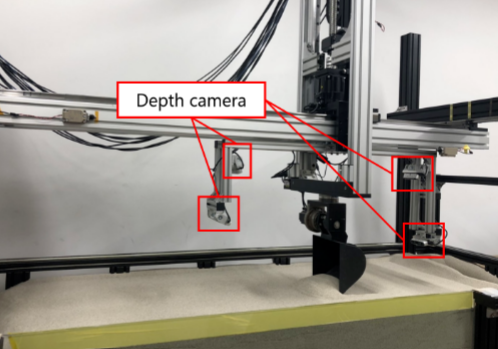
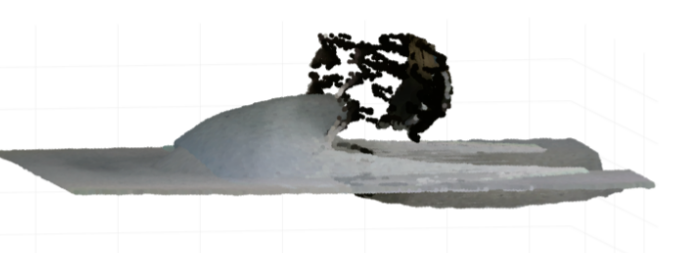
Excavation, which is indispensable for infrastructure construction, causes sand to collapse, so there are still many unknowns about the shape of the sediment during and after excavation. Therefore, the objective of this study was to predict sediment shape as accurately as possible by constructing a time-series prediction model. This study was able to adequately predict changes in sediment shape, which were not possible with the simplified models in the past terramechanics studies.
Development and improvement of a time-series prediction model of excavation resistance considering bucket trajectory and sediment geometry
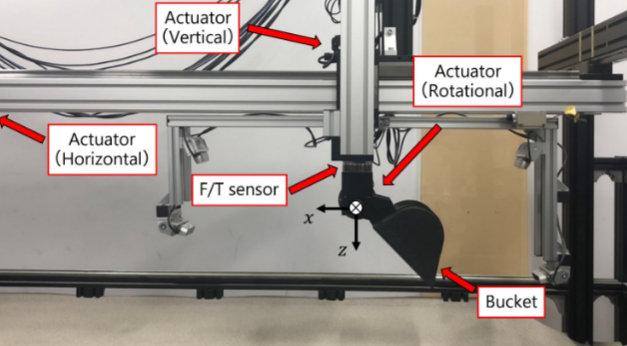
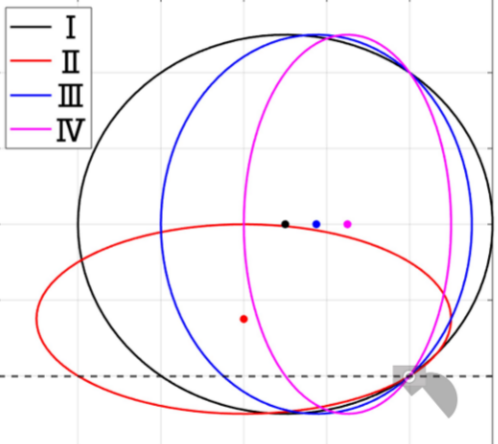
To avoid accidents involving construction equipment during excavation, the resistance of the bucket (the part that scoops up sand) must be properly evaluated. In this study, experiments were conducted using various bucket trajectories to predict excavation resistance. In most previous studies, the number of excavation experiments was limited to a single excavation from a horizontal sand surface, but the study predicted excavation resistance for multiple excavations to more closely simulate real-world conditions.
Construction of a workload prediction method for lunar work robots using transfer learning
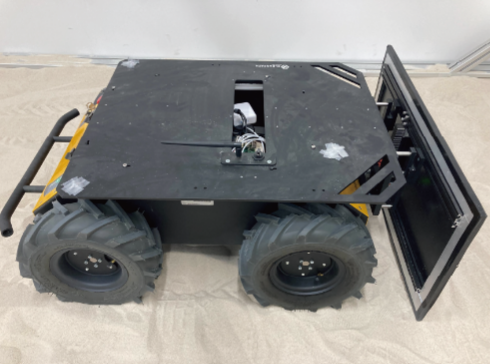
Predicting the workload is important for efficient work on the Moon. However, compared to work data on the ground, work data on the lunar surface is limited. Therefore, this study used transfer learning to predict the desired workload under the lunar environment based on ground-based work and some lunar surface data.
Thus, in infrastructure construction, such as excavation and soil drainage, our laboratory is working to predict unknown environmental conditions by using various machine learning methods for research areas that could not be predicted in past studies.